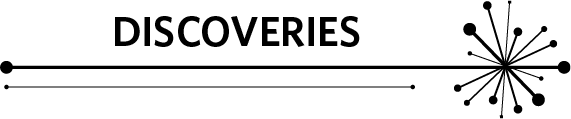
The Big Electric Brain
How machine learning allows U researchers to learn like, well... machines.
Satellites snap millions of images of virtually every acre of Earth, providing a potentially valuable database of shifting patterns of crops, grasslands, forest, and other land use. The information could help us understand changes in agriculture and the environment, as humans respond to a growing population and climate change.
But the amount of information is enormous—and growing daily. To categorize and make sense of it is a challenge. That’s where the University of Minnesota comes in. A handful of U researchers recently won a $1.43 million National Science Foundation grant to help figure out how to analyze the data.
Researchers from the College of Science and Engineering; the College of Food, Agricultural, and Natural Resource Sciences; and the Minnesota Supercomputing Institute (MSI) have proposed scanning, categorizing, and analyzing the images using machine learning, the next frontier of artificial intelligence, in which computer systems are able to train and teach themselves without being explicitly programmed.
In essence, these computer algorithms “have the ability to learn on their own,” says Mehmet Akçakaya, assistant professor in the Department of Electrical and Computer Engineering, who uses machine learning in a healthcare-related project. When shown new images, “they can learn what to do, essentially, just like us.”
Through machine learning, humans can analyze data that is so voluminous or complex that the task would take mere mortals ages—or it could not be done at all. U scientists are at the forefront of the revolution, using these algorithms for tasks ranging from predicting the likelihood of death to counting wildebeests. By integrating machine learning into research, scientific discoveries and advancements are taking the fast track.
The satellite imaging project will rely on machine learning’s ability to quickly scan and interpret images, says James Wilgenbusch, senior associate director of the MSI and a project coinvestigator.
The algorithm will “train” on the image database to distinguish forest from grassland, conifers from deciduous trees, corn from soybeans. The results will be incorporated into the U’s unique GEMS platform, which integrates genomic, environmental, management, and socioeconomic data to show, for example, how planting cover crops affects water quality.
“Those are practices that are difficult to actually monitor outside of survey data to determine whether there are positive benefits to certain types of practices,” says Wilgenbusch. “With the existing data we feel that we can do that.”
Patterns in human health
Nishant Sahni, M.D., a hospitalist and adjunct assistant professor in the Department of Medicine, recently collected electronic health records from nearly 60,000 hospitalizations. A “random forest” algorithm combed through data to determine the combinations of test results that predicted a patient had less than a year to live. Sahni wants to incorporate the program into electronic medical records to alert caregivers to “the highest-risk patients who might benefit from additional help,” including end-of-life counseling, or who might be spared unnecessary procedures.
“The next step is proving we can actually use the tool and do something with it clinically,” he says, “that we can intervene earlier, or have increased use of palliative care, improve our consultations, or increase patient satisfaction.”
Schooling researchers
Machine learning can identify hidden patterns in data that escape the notice of researchers, says Edward McFowland III, assistant professor of information and decision sciences in the Carlson School of Management.
A recent study showed that assigning a teacher’s aide to classrooms didn’t benefit elementary students. But when McFowland reexamined the data with a machine learning algorithm, it identified a subgroup of classrooms with highly experienced teachers that showed impressive gains. That pattern suggested several avenues of further research. “Machine learning has the ability to generate the hypotheses that ask the question that we don’t even know to ask.”
Deep learning in a heartbeat
A special category of machine learning is “deep learning,” where software learns to recognize patterns in sounds and images by mimicking the layers of neurons in the human brain. Deep learning is even more capable than standard machine learning of self-training.
Mehmet Akçakaya is training a deep-learning algorithm to speed up the acquisition of magnetic resonance imaging. Patients would benefit by spending less time in an MRI machine. Researchers could get accurate imaging of split-second events like a single heartbeat or the flash of a neuron. “You always want to go faster,” Akçakaya says. “If I can keep the person there for five minutes instead of 10 minutes, that’s going to reduce the cost. That’s going to reduce the patient discomfort. It’s going to be important both in the clinic and in research.”
Capturing motion
Through a deep-learning algorithm, Hyun Soo Park, an assistant professor in the Department of Computer Science and Engineering, is able to accurately track and analyze movements of human bodies, whether dribbling a basketball or swinging on a playground.
And he can do it without the strategically placed body markers that are used to make computer-generated imagery for movies, for example. Park has been able to do the same with free-roaming monkeys, which are widely used in studies to track the connection between brain signals and body movements. “Neuroscientists have been very interested in how their brain signals are correlated with their behavior,” says Park.
Counting wildebeests
Craig Packer, director of the U’s Lion Research Center, teamed up with a deep-learning expert to analyze the millions of camera-trap photos from his African field research. Packer relied on volunteers to read photos, but the volume became overwhelming.
The images are not nicely composed photos of easily recognized species. “Some of these brown antelope can look pretty similar to each other,” says Packer. “It could have its head down, facing the other direction. It does take an experienced person, if all they’re seeing is the rear end of a wildebeest, to say that’s a wildebeest. Likewise with a computer—if you put in enough examples of wildebeest pictures, all kinds of different angles, it can have the same kind of recognition capacity.”
The algorithm that Packer and his colleagues developed classifies and counts the species. “It’s really quite stunning in its abilities,” he says. “It’s something that will probably become integrated into almost every camera-trap study of any species anywhere on earth.”
Greg Breining (B.A. ’74) is a nature and science writer who lives in St. Paul. His latest book is Paddle North: Canoeing the Boundary Waters-Quetico Wilderness.